BN Speech Recognition
From HLT@INESC-ID
Automatic Speech Recognition (ASR) can be described as the process of machine transcriptioning into text the words uttered by human speakers. If one wants to perform a query in a database of BN data it is necessary to have a textual transcription or at least a detailed description of the news content.
State of the art ASR systems use hierarchical models to recognize speech. The input audio is broken into small chunks and the "acoustic model" of the ASR system attribute to each chunk a probability or likelihood of occurrence of a basic sound (phoneme). Then a search guided by two models determines the final sequence of words. The two models are the "lexicon" which models the sequence of phonemes that form words and the "language model" which models the sequence of words of the language.
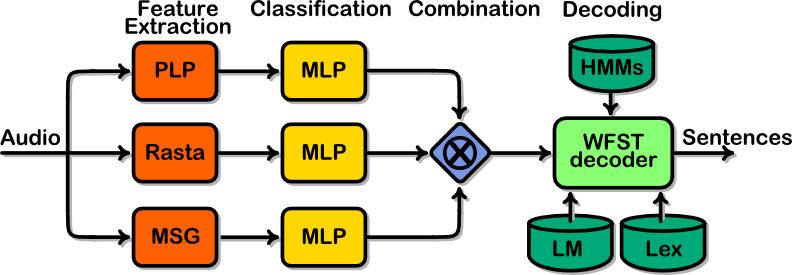
In the case of a BN task, training all these models involves large
amounts of data, both audio (for the "acoustic model") and text
(for the "language model"). Coping with large amounts of acoustic
data is an issue which L2F is addressing, more
specifically developing efficient training methods for the "acoustic model".